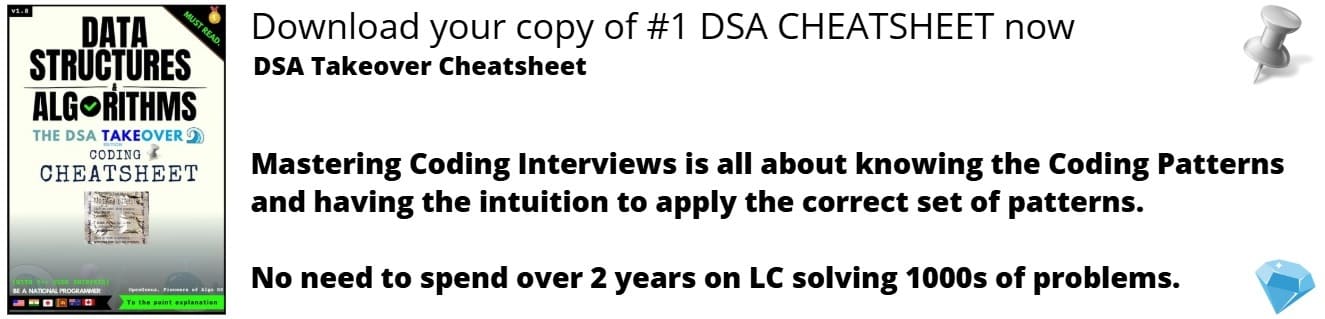
Open-Source Internship opportunity by OpenGenus for programmers. Apply now.
Divide and conquer optimization is used to optimize the run-time of a subset of Dynamic Programming problems from $O(N^2)$ to $O(N logN)$.
Quadrangle inequalities
For a two-variable function $f(x, y)$ :
-
Convex quandrangle inequality : $\forall_{a \leq b \leq c \leq d}(f(a, c) + f(b, d) \leq f(a, d) + f(b, c))$
-
Concave quadrangle inequality : $\forall_{a \leq b \leq c \leq d}(f(a, c) + f(b, d) \geq f(a, d) + f(b, c))$
Optimization criteria
A dynamic programming problem of the form:
$$
dp(i, j) = min_{k \leq j}(f(i, j, k))
$$
where,
$$
f(i, j, k) = dp(i - 1, k) + cost(k, j)
$$
Can be optimized using Divide and Conquer optimization if the function $cost(x, y)$ satisfies the convex-quadrangle inequality (sufficient but not necessary).
It can be noticed that the above recurrence, takes $O(n^3)$ time to be solved.
Note: Concave quadrangle inequality should be satisfied in case of maximization problem.
Optimization
If $cost(x, y)$ obey's the optimization criteria, it results in a useful property:
Note: In some cases even if the criteria is not satisfied, the following property can be observed and the optimization is still possible.
$$
h(i, j) \leq h(i, j + 1)
$$
where,
$$
h(i, j) = argmin_{k \lt j} (f(i, j, k))
$$
The above property can be generalized as,
$$
h(i, j^{\prime}) \leq h(i, j) \text{ , } j^{\prime} \lt j
$$
$h(x, y)$ is the smallest position where $dp(x, y)$ is optimal. This clearly tells us that the solution for $dp(x, y^{\prime})$ will always occur before the solution for $dp(x, y)$, where $y^{\prime} \lt y$ (monotonic).
Let,
$$
rec(x, yl, yr, kl, kr)
$$
be a function which recursively computes $dp(x, yl..yr)$ for a fixed $x$, given that the solution lies between $kl$ and $kr$.
This can be done by first computing $dp(x, mid)$ by iterating $k$ from $kl$ to $kr$, and then recursively calling
$$
rec(x, yl, mid - 1, kl, h(x, mid))
$$
and
$$
rec(x, mid + 1, yr, h(x, mid), kr)
$$
where, $mid = (yl + yr) / 2$.
The initial call will be $rec(x, 1, n, 1, n)$.
This works because, the solution for $dp(x, yl..mid - 1)$ will lie before the solution for $dp(x, mid)$ and the solution for $dp(x, mid + 1..yr)$ will lie after the solution for $dp(x, mid)$ because of the monotonicity of $h(x, y)$.
Complexity
For a fixed $x$, the number of operations of the above function $rec$ will be of the form:
$$
T(n) = 2T(n/2) + O(n)
$$
Because of two recursive calls, each with half of the original problem size and another $O(n)$ for finding the solution for the $mid$. By master's theorem, the function will have a complexity of $O(nlogn)$.
Overall compexity will be $O(knlogn)$, because $x$ can take values from 0 to $k-1$.
Example to demonstrate Divide and Conquer Optimization
Problem
There are $p$ people at an amusement park who are in a queue for a ride. Each pair of people has a measured level of unfamiliarity. The people will be divided into $g$ non-empty contiguous groups. Each division has a total unfamiliarity value which is the sum of the levels of unfamiliarity between any pair of people for each group. Determine the minimal possible total unfamiliarity value.
Given a $pxp$ matrix $unfamiliarity$, where $unfamiliarity[x][y]$ is the measure of unfamiliarity between person $x$ and $y$, find the minimum possible total unfamiliarity after dividing the $p$ people into $g$ non-empty contiguous groups. ($unfamiliarity[x][y] = unfamiliarity[y][x]$)
Example: If there are 3 ($p$) people and they have to be divided into 2 non-empty contiguous groups ($g$) where unfamiliarity between person 0 and 1 is 2 ($unfamiliarity[0][1] = unfamiliarity[1][0] = 2$), between person 1 and 2 is 3 ($unfamiliarity[1][2] = unfamiliarity[2][1] = 3$) and between person 0 and 2 is 0 ($unfamiliarity[0][2] = unfamiliarity[2][0] = 0$).
Minimal unfamiliarity of value 2 is obtained when 0 and 1 is present in one group and 2 is present in the otherjj.
The only other division into 2 non-empty contiguous groups is {{0}, {1, 2}} and that has a total unfamiliarity of 3.
Solution
Let $cost(l, r)$ be the unfamiliarity of a contiguous group from $l$ to $r$ (that is the value if all the people from $l$ to $r$ are grouped together). $cost(l, r)$ can be found in constant time by finding the two-dimensional prefix sum matrix associated with the $unfamiliarity$ matrix.
State: Let $dp[x][y]$ represent the minimum unfamiliarity when the people $1..y$ are split into $x$ groups.
Transition: To compute $dp[x][y]$, the position where the $x$-th contiguous group should start is required. This can be found by iterating $k$ from $0..y$ and computing the unfamiliarity by cutting at each $k$:
$$
dp[x][y] = min_{0 \leq k \lt y}(dp[x - 1][k] + cost(k + 1, y))
$$
Unoptimized implementation:
const int P = MAX_PEOPLE;
const int G = MAX_GROUPS;
int pre[P][P];
int dp[G][P];
int cost(int l, int r) {
--l;
return pre[r][r] - pre[l][r] - pre[r][l] + pre[l][l];
}
void prefixSum(vector<vector<int>>& unfamiliarity) {
for(int i = 1; i < unfamiliarity.size(); ++i) {
for(int j = 1; j < unfamiliarity.size(); ++j) {
pre[i][j] = unfamiliarity[i][j] + pre[i - 1][j] + pre[i][j - 1] - pre[i - 1][j - 1];
}
}
}
int rec(int x, int y) {
if(x == 0 && y == 0) { return 0; }
if(x == 0) { return (1 << 30); }
int& xy = dp[x][y];
if(xy != -1) { return dp[x][y]; }
xy = (1 << 30);
for(int k = 0; k < y; ++k) {
xy = min(xy, rec(x - 1, k) + cost(k + 1, y));
}
return xy;
}
int minimumUnfamiliarity(int p, int g, vector<vector<int>> unfamiliarity) {
prefixSum(unfamiliarity);
memset(dp, -1, sizeof dp);
return rec(g, p) / 2;
}
The above solution uses prefixSum
function to find the prefix sum of unfamiliarity
and the rec
function to find the minimal unfamiliarity after dividing into g
contiguous sequences.
Notice that the cost
function satisfies the convex-quadrangle inequality (because it's based on prefix sums). So the above implementation can be optimized using divide and conquer.
const int P = MAX_PEOPLE;
const int G = MAX_GROUPS;
int pre[P][P];
int dp[G][P];
int cost(int l, int r) {
--l;
return pre[r][r] - pre[l][r] - pre[r][l] + pre[l][l];
}
void prefixSum(vector<vector<int>>& unfamiliarity) {
for(int i = 1; i < unfamiliarity.size(); ++i) {
for(int j = 1; j < unfamiliarity.size(); ++j) {
pre[i][j] = unfamiliarity[i][j] + pre[i - 1][j] + pre[i][j - 1] - pre[i - 1][j - 1];
}
}
}
int rec(int x, int yl, int yr, int kl, int kr) {
if(yl > yr) { return 0; }
int mid = yl + (yr - yl) / 2;
int pos = 0;
dp[x][mid] = 1 << 30;
for(int k = kl; k <= min(mid - 1, kr); ++k) {
int cur = dp[x - 1][k] + cost(k + 1, mid);
if(cur < dp[x][mid]) {
dp[x][mid] = cur;
pos = k;
}
}
rec(x, yl, mid - 1, kl, pos);
rec(x, mid + 1, yr, pos, kr);
}
int minimumUnfamiliarity(int p, int g, vector<vector<int>> unfamiliarity) {
prefixSum(unfamiliarity);
for(int i = 0; i < P; ++i) { dp[0][i] = 1 << 30; }
dp[0][0] = 0;
for(int x = 1; x <= g; ++x) {
rec(x, 1, p, 0, p - 1);
}
return dp[g][p] / 2;
}
This implementation, divides the problem into two-equal halves as described before. The function rec
computes $dp(x, yl..yr)$ for a fixed x by recursively computing for the left and right halves of $yl$ to $yr$ after finding $dp(x, mid)$ - dp[x][mid]
and $h(x, mid)$ - pos
(position where $dp(x, mid)$ is minimum). The function minimumUnfamiliarity
makes a call to rec
for every value of x
.
Reducing the complexity from $O(kn^2)$ to $O(knlogn)$.
Question
What is the space complexity of minimumUnfamiliarity?
With this article at OpenGenus, you must have the complete idea of Divide and Conquer Optimization in Dynamic Programming. Enjoy.