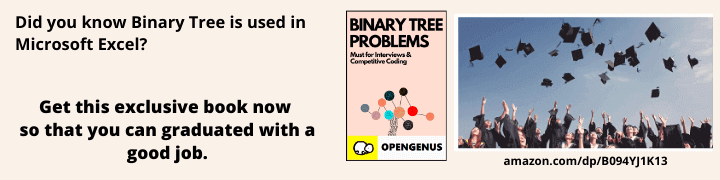
Open-Source Internship opportunity by OpenGenus for programmers. Apply now.
Index:
- Introduction
- Understanding Correlation
- Understanding Causation
- The Dangers of Mixing Correlation and Causation
- Real-World Examples
- How to Distinguish Correlation from Causation
- Conclusion
- References
Introduction:
The ideas of correlation and causation are essential for understanding data and coming to conclusions. This article at OpenGenus will examine the definitions of these phrases, the reasons why they are frequently misinterpreted, and how to tell them apart. The following article is for all interested in learning about the world of data, whether they are a fan of computer science, a data analyst, or just curious.
Understanding Correlation:
A mathematical measure known as correlation quantifies how much two variables change together over time. It does not imply causation, that is just because two variables have a correlation does not mean one of them is the cause of the other. There are two types of correlation: positive and negative. A positive correlation occurs when both variables rise or fall together, while a negative correlation occurs when one variable rises as the other falls.
Understanding Causation:
Causation suggests that alterations in one variable have an immediate effect on the other variable. Studies that are controlled and a deeper comprehension of the underlying systems are frequently needed to prove causation. Correlation is much simpler to establish than causality.
The Dangers of Mixing Correlation and Causation:
Making the mistake of confusing correlation with causation might result in incorrect conclusions. For instance, just because ice cream sales and drownings both rise in the summer does not always imply that consuming ice cream causes drownings. A third component, hot weather, has an effect on both variables.
Real-World Examples:
Let's look at some examples of correlation and causation in the real world:
-
Correlation: The number of firemen on the site and the extent of the fire's destruction are positively correlated.
Causation: Hiring more firemen does not make fires more destructive; rather, when a fire is severe, more firefighters are dispatched. -
Correlation: The sales of umbrellas and ice cream cones at a beachside kiosk are positively correlated. When umbrella sales go up, ice cream sales also increase.
Causation: It's not the sale of umbrellas that causes an increase in ice cream sales or vice versa. Instead, both are driven by the weather. On a hot, sunny day, people buy more ice cream and umbrellas to shield themselves from the sun. The common cause here is the weather, not one product causing an increase in the other. -
Correlation: The quantity of winter coats sold and the quantity of sunglasses sold are inversely correlated.
Causation: Winter coat sales are affected by the seasons, not by people buying more sunglasses.
How to Distinguish Correlation from Causation:
• Examine the context: Recognize the setting in which data was obtained. Search for potential confounding elements that might account for the link that was noticed.
• Take into account temporal order: If one event is causing another, it should come first in the timeline.
• Experimentation: Controlled experiments are frequently required to prove causation. Changing one variable while holding the others constant will allow you to see how it affects the other.
• Apply domain knowledge: Make use of your knowledge of the topic to see whether a causal relationship makes sense.
Conclusion:
Making the distinction between correlation and causation is crucial when it comes to data analysis and decision-making. Although correlation might offer insightful information, it is causation that enables us to come to conclusions, make predictions, and take significant action. Simply because two events seem to be connected doesn't necessarily mean that one is the root of the other. To make better conclusions, approach data analysis cautiously and with an understanding of the nuances of these ideas.
References:
- Palmer-Jones, C., & Lee, J. C. (2023). Mistakes in biomarkers for IBD and how to avoid them.
- Hume, D. (2016). An enquiry concerning human understanding. In Seven masterpieces of philosophy (pp. 183-276). Routledge.