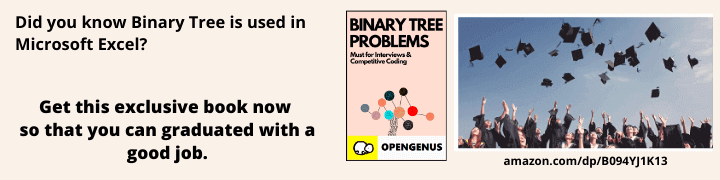
Open-Source Internship opportunity by OpenGenus for programmers. Apply now.
Quantum computing has the potential to transform numerous industries, including encryption and data security. While typical computers store and process information using binary digits, or bits, quantum computers use quantum bits, or qubits, which can exist in several states at the same time. As a result, quantum computers may do some calculations significantly quicker than ordinary computers, such as those used in encryption and data security.
The influence of quantum computing on cryptography and data security, on the other hand, is complex and diverse. Here are some of the major impacts that quantum computing is projected to have on various fields:
- Breaking Cryptographic Systems
- Developing Quantum-Safe Cryptography
- Improving Cybersecurity
- Advancing Cryptographic Research
- Enhancing Privacy
- Accelerating Drug Discovery
- Personalizing Treatment Plans
- Improving Medical Imaging
- Streamlining Administrative Tasks
- Enhancing Disease Surveillance
We will dive into each point now.
Breaking Cryptographic Systems
One of the most significant possible effects of quantum computing on cryptography is the capacity to break many of the current encryption schemes. The widely used RSA and Elliptic Curve Cryptography (ECC) techniques, for example, are both vulnerable to quantum computer assaults. This is due to quantum computers' ability to execute Shor's algorithm calculations, which can swiftly factor enormous numbers, allowing them to break many encryption keys.
Developing Quantum-Safe Cryptography
Researchers are working on building quantum-safe or post-quantum cryptography in response to the threat posed by quantum computers to standard encryption technologies. These new encryption methods are meant to be immune to attacks by both classical and quantum computers, preserving data security even as quantum computing progresses.
Improving Cybersecurity
Quantum computing can also be used to improve cybersecurity by more rapidly and effectively identifying and responding to cyber attacks. Quantum computing, for example, can be used to perform more complicated and precise threat modelling, allowing cybersecurity professionals to identify and respond to possible threats faster. Furthermore, quantum computing may be used to analyse massive amounts of data, allowing cybersecurity professionals to detect and respond to cyber assaults faster and more efficiently.
Advancing Cryptographic Research
Quantum computing can also be utilised to boost cryptographic research by enabling researchers to create new and more efficient cryptographic methods. Quantum computing, for example, can be used to model the behaviour of atoms and molecules, allowing researchers to better understand fundamental quantum mechanics principles and build new cryptographic algorithms based on these concepts.
Enhancing Privacy
Quantum computing can potentially be used to improve privacy by enabling the creation of more secure data transport and storage technologies. Quantum key distribution (QKD), for example, uses quantum physics to ensure the secure transmission of encryption keys. QKD employs quantum entanglement qualities to ensure that any effort to intercept the transmission of the encryption key is quickly recognised, assuring the key's security.
Accelerating Drug Discovery
By analysing enormous datasets of chemical and biological information, machine learning algorithms can be utilised to speed up the drug discovery process. Machine learning algorithms, for example, can be used to predict the efficacy of novel treatments and identify probable adverse effects, saving time and money over traditional drug discovery approaches. Machine learning algorithms can also be used to analyse genetic data and find possible pharmacological targets, enabling the development of more tailored and effective medicines.
Personalizing Treatment Plans
Machine learning algorithms can also be utilised to create patient-specific treatment strategies. Machine learning algorithms can assist physicians in identifying the most successful treatments for specific patients by analysing enormous volumes of data such as medical history, genetics, and environmental factors. By minimising the need for trial-and-error treatment procedures, this can help to improve patient outcomes and save healthcare expenditures.
Improving Medical Imaging
By analysing massive datasets of medical images and discovering patterns that may not be evident to the human eye, machine learning algorithms can be used to improve medical imaging. Machine learning algorithms, for example, can be used to detect early indicators of disease in medical imaging, allowing for earlier intervention and treatment. Furthermore, machine learning techniques can be utilised to improve medical imaging accuracy by reducing errors and enhancing efficiency.
Streamlining Administrative Tasks
Machine learning algorithms can also be used to improve the patient experience by streamlining administrative activities in healthcare. Machine learning algorithms, for example, can be used to automate chores like appointment scheduling and billing, freeing up healthcare workers to focus on patient care. Furthermore, machine learning algorithms can be used to improve the accuracy and efficiency of medical coding, reducing errors and enhancing compensation for healthcare professionals.
Enhancing Disease Surveillance
By analysing huge volumes of data and discovering patterns that may suggest the beginning of a new disease outbreak, machine learning algorithms can be used to improve disease surveillance. Machine learning algorithms, for example, can be used to analyse social media data and detect early warning indications of disease outbreaks, allowing for earlier intervention and control measures. Furthermore, machine learning algorithms can be used to analyse electronic health information and identify possible disease clusters, enabling more targeted surveillance and intervention efforts.
Conclusion
To summarise this article at OpenGenus, the advantages of employing machine learning in healthcare are substantial and far-reaching. Among other things, machine learning algorithms can be used to improve patient outcomes, lower healthcare expenditures, and improve disease surveillance. As the field of machine learning evolves, it will be critical for healthcare professionals and researchers to collaborate to ensure that the potential benefits of machine learning are realised while minimising the risks and ethical concerns associated with its use.